Rigid Motion Gaussian Processes with SE(3) Kernel and Application to Visual Pursuit Control
Abstract:
We address in this letter the learning of unknown rigid body motions in the Special Euclidian Group SE(3) based on Gaussian Processes. A new covariance kernel for SE(3) is presented and proven to be a valid kernel for Gaussian Process Regression. The learning error of the proposed Gaussian Process model is extended to a highprobability statement on SE(3). We employ it in a visual pursuit scenario of a moving target with unknown velocity in 3D space. Our approach is validated in a simulated 3D environment in Unity, and shows significant better prediction accuracy than the most commonly used Gaussian kernel. When compared to other covariance kernels proposed on SE(3), its advantages are a natural extension of covering numbers to SE(3), that it is computationally more efficient, and that stability of target pursuit can be guaranteed without limiting the target rotational space to SO(2).
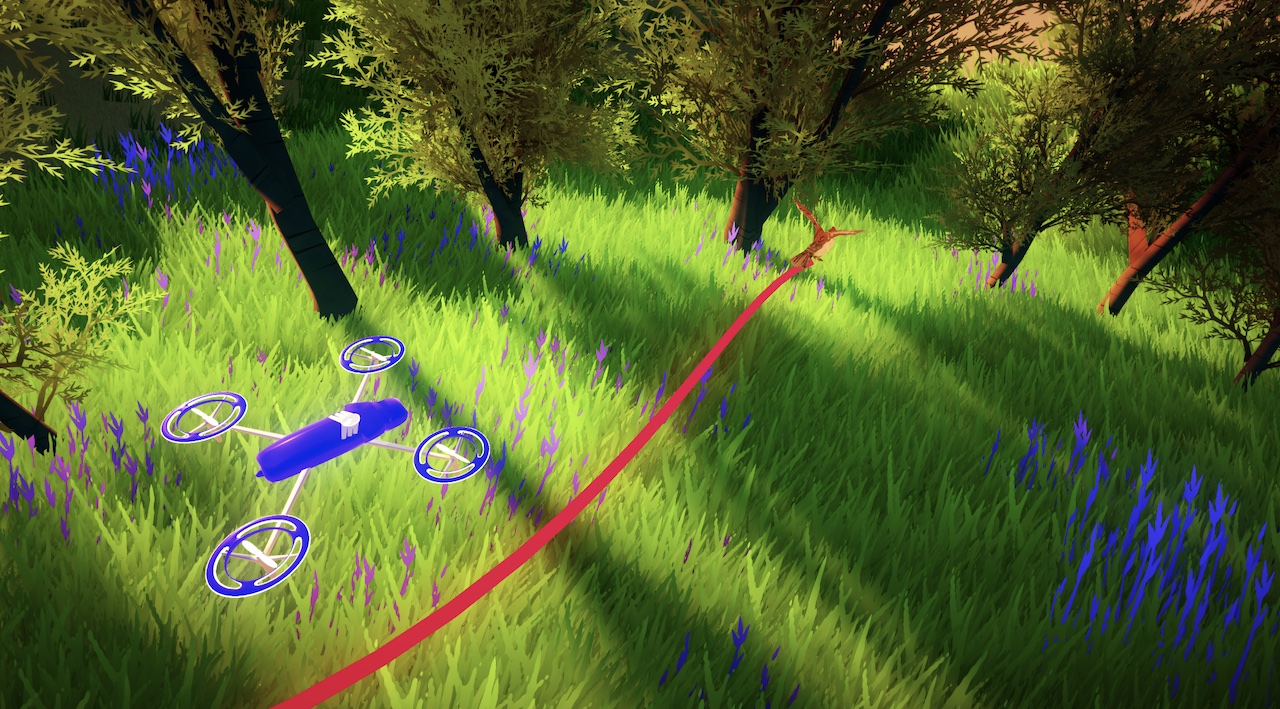
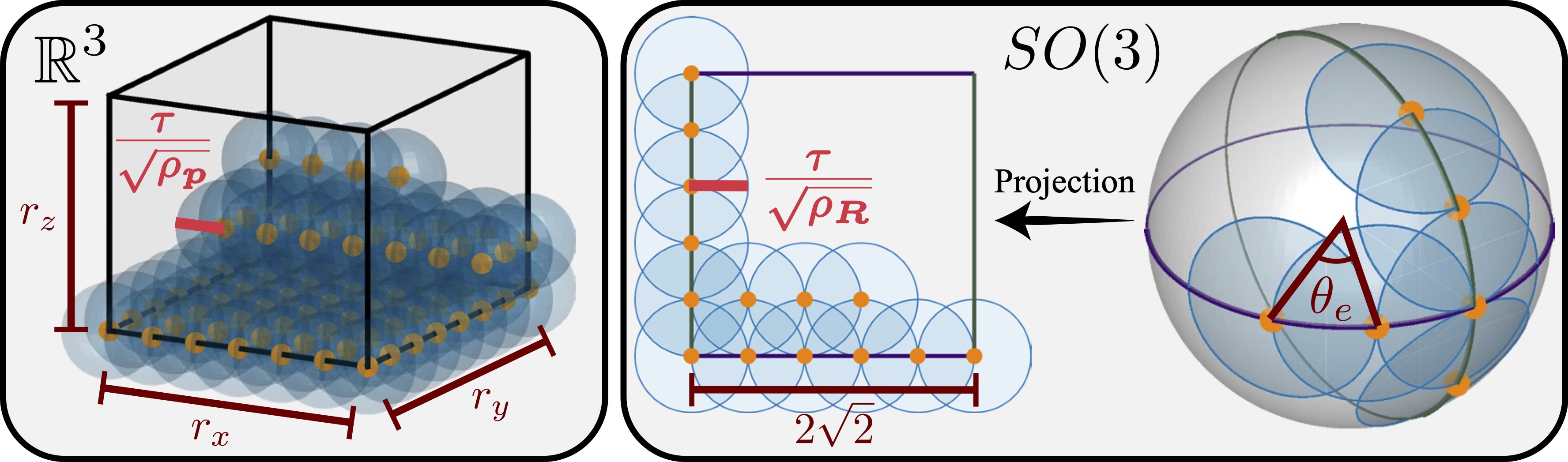
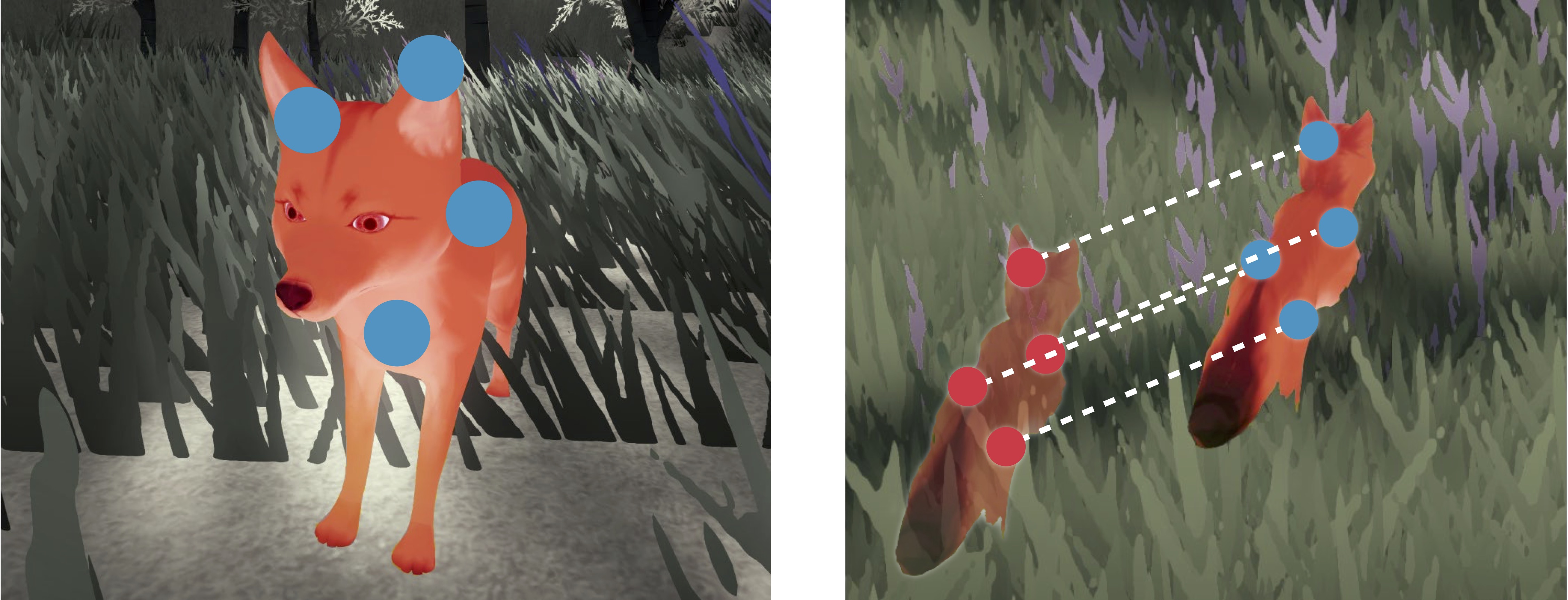
Comments